Why Next-Gen Analytics Requires Robust Data Quality Monitoring
April 3, 2023
Originally published on the Google blog
We often hear that being data-driven is essential for organizations. But there’s something missing from this statement. You need to be driven by high-quality data — or else you might be driving in the entirely wrong direction.
Google BigQuery unlocks powerful next-generation analytics and ML applications. To use BigQuery with high-quality data, you need a comprehensive data quality monitoring solution that alerts you of unexpected changes in the data itself and pinpoints the reasons behind those changes.
In this article, we’ll show you how to instill trust in your next-generation analytics strategy by setting up a comprehensive data quality monitoring strategy with BigQuery and Anomalo.
How data issues can affect the quality of your analytics
With a fully managed data warehouse like BigQuery, you can quickly spin up a modern data stack that supports a variety of analytics use cases. However, just as it’s easy to take advantage of more data sources and volume than ever before, it also leaves more scope for data issues. Unexpected changes in data values is a deep issue that’s particularly difficult to detect and understand, since there are often many possible explanations to evaluate. Was a spike in purchases caused by an error somewhere in your data pipeline, or by a seasonal trend? Is your ML model performing poorly because its input data has suddenly changed, or because there was a flaw in the model design?
Without confidence in the data quality, we see that teams face problems such as:
- An executive notices some unusual numbers on a dashboard and suspects that there is a data quality bug, causing a fire drill for the analytics/data engineering teams as they try to find the root cause.
- An ML model starts performing erratically, affecting customers, because the distribution of the production data has drifted from the distribution of the training data without anyone on the team being alerted to the change.
In early 2022, there was a high-profile example of what can go wrong when Equifax reported millions of incorrect credit scores. Lacking the automated data quality needed to detect the issue, financial institutions used this data in production and ended up denying loans to qualified individuals.
With comprehensive data quality, you can detect complex issues at scale
Enterprises need data quality tools that can help them detect and resolve complicated data issues, before issues affect BI dashboards and reports or downstream ML models. These tools can answer questions like:
- Is my data correct and consistent, based on the recent past and subject matter experts’ expectations of what the data should look like?
- Are there significant changes in my metrics?
- Why is my data unexpectedly changing? What is causing these issues?
- Are my ML inputs drifting? Why?
Foundational data observability includes a number of basic tests, such as whether data pipelines completed successfully, or whether the volume of data ingested was in line with expectations. These tests focus more on the process than on the data itself. On the other hand, comprehensive data quality monitoring goes beyond basic observability checks and looks at the actual contents of the data. It helps with the hardest parts of data quality, like tracking data drift and monitoring metrics changes.
Dataplex is an intelligent data fabric that provides a way to manage, monitor, and govern your distributed data at scale. Dataplex provides two options to validate data quality: Auto data quality (Public Preview) & Dataplex data quality task. Dataplex AutoDQ and data quality now enable next-generation data quality solutions that automate rule creation and at-scale deployment of data quality. We also work with our partners like Anomalo with richer features to provide solutions completeness to our customers.
Go beyond basic data observability with BigQuery and Anomalo
Anomalo, a Google Cloud Ready – BigQuery partner, is a comprehensive data quality monitoring platform that plugs directly into your data stack. Anomalo’s deep data quality goes beyond checks like data freshness to automatically detect key metrics changes and table anomalies. Below, you can see how Anomalo is deployed on GCP and integrates with BigQuery and other services to identify customer’s data quality issues:
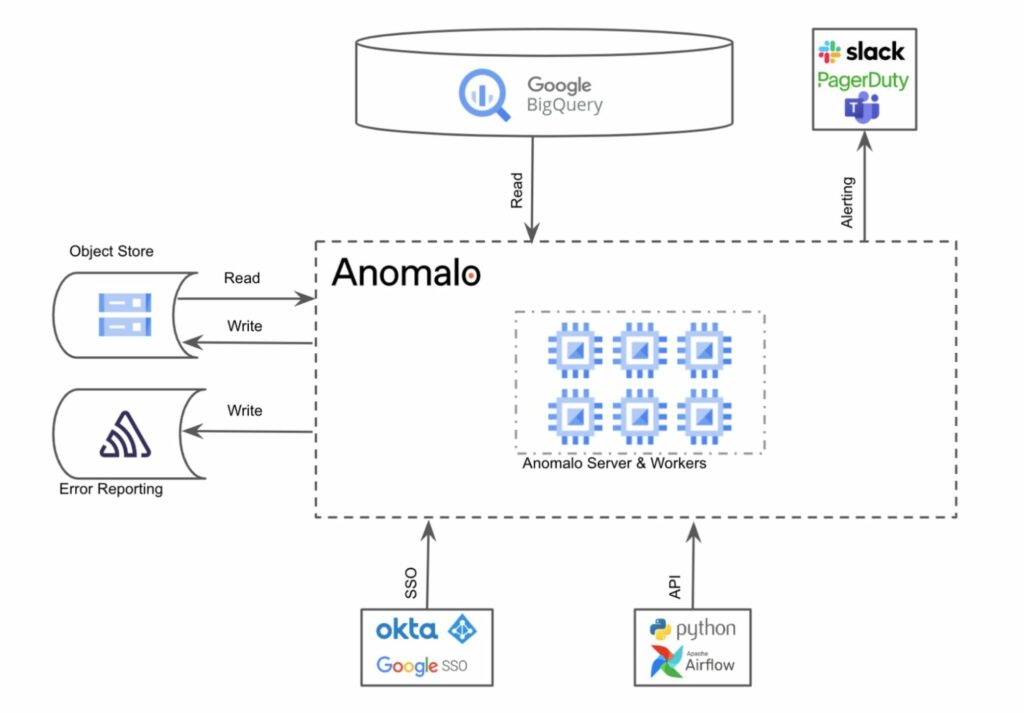
With continuous monitoring, you have peace of mind that your data is always accurate even as it evolves over time. The platform’s no-code UI makes it easy for anyone to be a data steward or consumer.
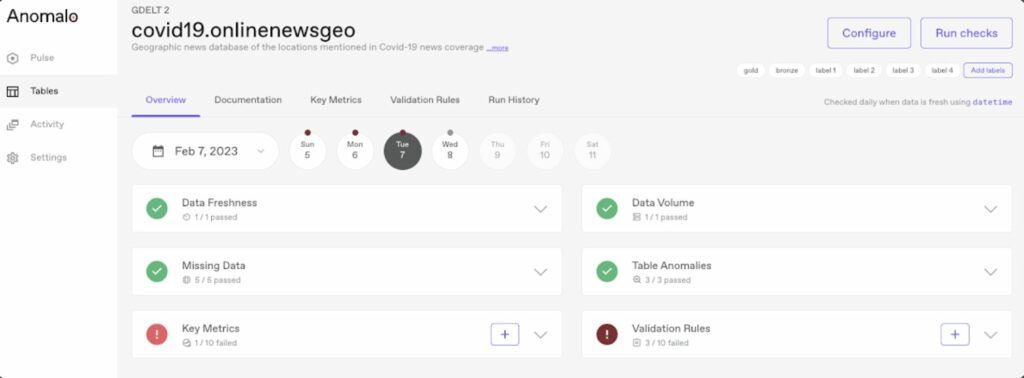
Anomalo provides rich, visual alerts that integrate with Slack and Gmail. Here’s an example of how Anomalo can let you know when data falls outside of an expected range. Observers get a quick overview of how unusual the data is compared to the norm, and can drill deeper for a complete breakdown of the data factors that contributed to the deviation.
Alert in Slack:
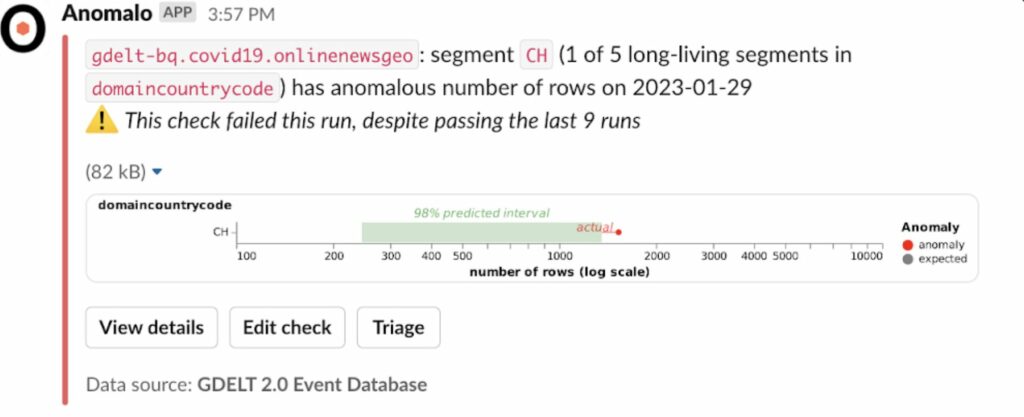
Expanded root cause analysis in Anomalo:
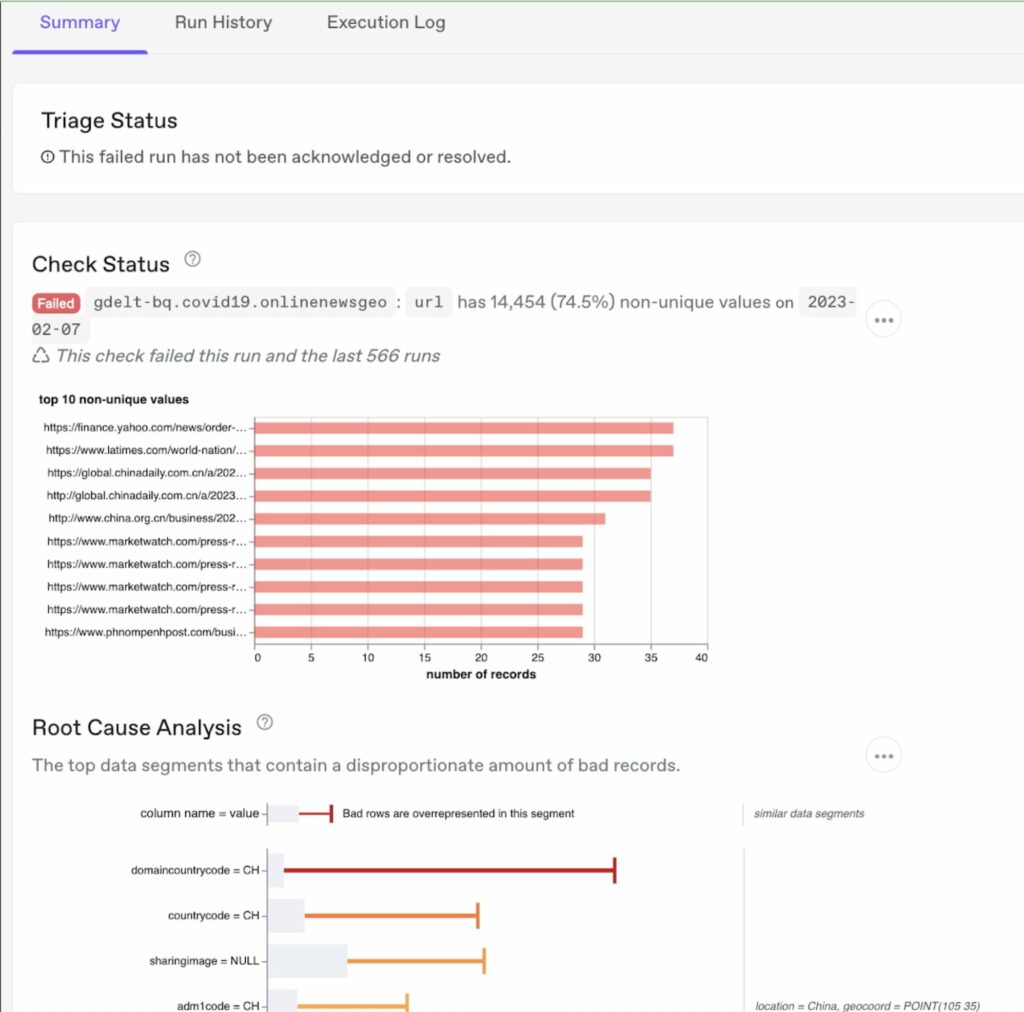
Anomalo supports a one-click integration with BigQuery. Simply enter a few details about your account, and Anomalo will automatically start providing both data observability and automated data quality for all of your selected tables.
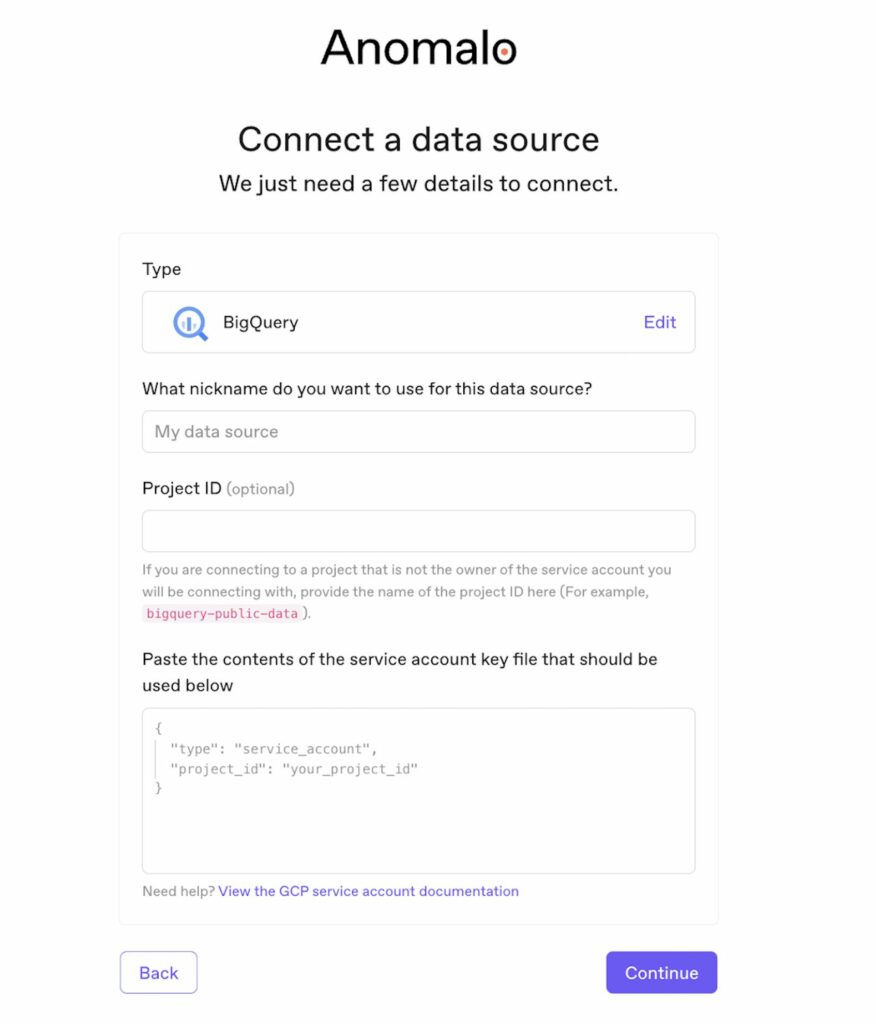
In conclusion
BigQuery offers unprecedented scalability and speed for your analytics needs. As you use these capabilities to do more with your data, it’s important to have confidence that the data itself is high quality. Leveraging data insights without automated data quality can expose your business, products, and users to unwanted risk. When quality is in place, not only is risk minimized, but everyone knows they can trust the data, increasing the adoption of analytics across the organization.
With Anomalo, BigQuery users can monitor for data issues and resolve them quickly. Click here to learn more about the BigQuery and Anomalo partnership. Click here to learn more about Anomalo.
Get Started
Meet with our expert team and learn how Anomalo can help you achieve high data quality with less effort.